Difference between revisions of "20.109(S21):M3D3"
Noreen Lyell (Talk | contribs) (→Part 3: Purify IPC protein) |
Noreen Lyell (Talk | contribs) |
||
Line 5: | Line 5: | ||
==Introduction== | ==Introduction== | ||
− | + | As evidenced by Nagai’s work, wild-type inverse pericam is not toxic to BL21(DE3)pLysS cells. Although it is unlikely that your point mutation will dramatically change this fact, in general a novel protein may turn out to be toxic. If this is the case, only very small amounts of protein are produced before the bacteria die. Keep in mind that overexpressing a single protein may come at the expense of producing proteins needed for survival, and will most likely cause cell death eventually; however, toxic proteins hasten this demise. Aberrant toxicity can sometimes be alleviated by reducing the culture temperature (e.g., to 30 °C). | |
− | + | Based on its fluorescence activity, wild-type inverse pericam allows proper folding of (cp)EYFP, and based on its response to calcium, it also allows calmodulin to fold. One problem you may encounter is that your mutant proteins will no longer fold correctly. Since you made mutations in the calcium sensor part of IPC, rather than the fluorescent part, it is unlikely that your protein will destroy EYFP fluorescence. However, a common problem with misfolded proteins is the formation of insoluble aggregates, due for instance to improperly exposed hydrophobic surfaces. Proteins can be purified from these aggregates – called inclusion bodies – but the process is more labor-intensive than for soluble proteins. (The proteins must be extracted under more harsh conditions than you will use next time, then purified under denaturing conditions, before finally attempting to renature the proteins.) Inclusion bodies sometimes form simply due to very high expression of the protein of interest, causing it to pass its solubility limit. This outcome can be prevented by lowering the culture temperature, the induction duration, the amount of IPTG, or the growth phase of the bacteria. | |
− | + | One final point to keep in mind is that not all proteins can be produced in bacteria. Eukaryotic proteins that require post-translational modifications (such as glycosylation) for activity require eukaryotic hosts (such as yeast, or the commonly used CHO – Chinese hamster ovary – cells). Sometimes eukaryote-derived proteins will be truncated or otherwise mistranslated by E. coli due to differential codon bias; errors in translation can be prevented by providing additional tRNAs to the culture or directly to the bacteria via plasmids. Despite all this complexity, prokaryotic hosts have been plenty good enough to produce proteins for certain therapies, notably the cytokine G-CSF. This cytokine is taken by patients needing to replenish their white blood cells (e.g., after chemotherapy), and sold as Neupogen by the company Amgen. | |
− | |||
− | + | This is it, folks! Moment of truth. Time to find out how the proteins that you worked so hard to express, purify, and test really behave. Although you should be able to produce reasonable titration curves by following the example of Nagai, the introduction/review of binding constants below may help contextualize your analysis. | |
− | + | Let’s start by considering the simple case of a receptor-ligand pair that are exclusive to each other, and in which the receptor is monovalent. The ligand (L) and receptor (R) form a complex (C), which can be written | |
− | + | <center> | |
+ | <math> R + L \rightleftharpoons\ ^{k_f}_{k_r} C </math> | ||
+ | </center> | ||
− | === | + | At equilibrium, the rates of the forward reaction (rate constant = <math>k_f</math>) and reverse reaction (rate constant = <math>k_r</math>) must be equivalent. Solving this equivalence yields an equilibrium dissociation constant <math>K_d</math>, which may be defined either as <math>k_r/k_f</math>, or as <math>[R][L]/[C]</math>, where brackets indicate the molar concentration of a species. Meanwhile, the fraction of receptors that are bound to ligand at equilibrium, often called ''y'' or θ, is <math>C/R_{TOT}</math>, where <math>R_{TOT}</math> indicates total (both bound and unbound) receptors. Note that the position of the equilibrium (''i.e.'', ''y'') depends on the starting concentrations of the reactants; however, <math>K_d</math> is always the same value. The total number of receptors <math>R_{TOT}</math>= [''C''] (ligand-bound receptors) + [''R''] (unbound receptors). Thus, |
− | + | <center> | |
+ | <math>\qquad y = {[C] \over R_{TOT}} \qquad = \qquad {[C] \over [C] + [R]} \qquad = \qquad {[L] \over [L] + [K_d]} \qquad</math> | ||
+ | </center> | ||
− | + | where the right-hand equation was derived by algebraic substitution. If the ligand concentration is in excess of the concentration of the receptor, [''L''] may be approximated as a constant, ''L'', for any given equilibrium. Let’s explore the implications of this result: | |
− | + | *What happens when ''L'' << <math>K_d</math>? | |
+ | ::→Then ''y'' ~ <math>L/K_d</math>, and the binding fraction increases in a first-order fashion, directly proportional to ''L''. | ||
− | + | *What happens when ''L'' >> <math>K_d</math>? | |
+ | ::→In this case ''y'' ~1, so the binding fraction becomes approximately constant, and the receptors are saturated. | ||
− | < | + | *What happens when ''L'' = <math>K_d</math>? |
− | + | ::→Then ''y'' = 0.5, and the fraction of receptors that are bound to ligand is 50%. This is why you can read <math>K_d</math> directly off of the plots in Nagai’s paper (compare Figure 3 and Table 1). When ''y'' = 0.5, the concentration of free calcium (our [''L'']) is equal to <math>K_d</math>. '''This is a great rule of thumb to know.''' | |
− | + | The figures below demonstrate how to read <math>K_d</math> from binding curves. You will find semilog plots (right) particularly useful today, but the linear plot (left) can be a helpful visualization as well. Keep in mind that every ''L'' value is associated with a particular equilbrium value of ''y'', while the curve as a whole gives information on the global equilibrium constant <math>K_d</math>. | |
− | + | [[Image:20109 Fa15 M2D7 figure.png|thumb|300px|left|'''Simple binding curve.''' The binding fraction ''y'' at first increases linearly as the starting ligand concentration is increased, then asymptotically approaches full saturation (''y''=1). The dissociation constant <math>K_d</math> is equal to the ligand concentration [''L''] for which ''y'' = 1/2.]] | |
+ | [[Image:20109 Fa15 M2D7 figure2.png|thumb|300px|center|'''Semilog binding curves.''' By converting ligand concentrations to logspace, the dissociation constant is readily determined from the inflection point of the sigmoidal curve. The three curves each represent different ligand species. The middle curve has a <math>K_d</math> close to 10 nM, while the right-hand curve has a higher <math>K_d</math> and therefore lower affinity between ligand and receptor (vice-versa for the left-hand curve).]] | ||
+ | <br style="clear:both;"/> | ||
− | + | Of course, inverse pericam has multiple binding sites, and thus IPC-calcium binding is actually more complicated than the example above. The <math>K_d</math> reported by Nagai is called an ‘apparent <math>K_d</math>’ because it reflects the overall avidity of multiple calcium binding sites, not their individual affinities for calcium. Normally, calmodulin has a low affinity (N-terminus) and a high affinity (C-terminus) pair of calcium binding sites. However, the E104Q mutant, which is the version of CaM used in inverse pericam, displays low affinity binding at both termini. Moreover, the Hill coefficient, which quantifies cooperativity of binding in the case of multiple sites, is reported to be 1.0 for inverse pericam. This indicates that inverse pericam behaves as if it were binding only a single calcium ion per molecule. Thus, wild-type IPC is well-described by a single apparent <math>K_d</math>. | |
− | + | For any given mutant, things may be more complicated. Keep in mind that we are not directly measuring calcium binding, but instead are indirectly inferring it based on fluorescence (for both mutant and wild-type IPC). A change in fluorescence requires the participation not only of calcium, but also of M13. In addition to the four separate calcium binding sites in calmodulin, the M13 binding site influences apparent affinity and apparent cooperativity. In short, be careful about how you describe the meanings of our binding parameters in your reports. | |
− | + | ||
− | + | ||
− | + | ||
− | + | ||
− | + | ||
− | + | ||
− | + | ||
− | + | Returning to the big picture: when you write your Protein engineering summary, be sure to consider how changes in both binding affinity and cooperativity (and even potentially raw fluorescence differences) can affect the practical utility of a sensor. | |
− | + | ||
− | + | ==Protocols== | |
− | + | ===Part 2: Prepare samples for titration curve=== | |
− | + | ||
− | + | ||
− | + | ||
− | + | ||
− | + | ||
− | + | ||
− | + | ||
− | + | ==== Tips for success==== | |
− | + | Take great care today to limit the introduction of bubbles in your samples. When expelling fluid, pipet '''''slowly''''' while touching the pipet tip against the bottom or side of the well. | |
+ | <!-- | ||
+ | When using the multichannel pipet, always check to make sure all tips are getting filled - sometimes one tip may not be on all the way, and will pull up less volume than the others. If this happens, release the fluid, adjust the tip, and try again. | ||
+ | --> | ||
− | + | ====Protocol==== | |
− | + | ||
− | + | ||
− | + | ||
− | + | [[File:Fa15 Protein Ca assay plate map.png|thumb|450px|right|Titration plate map]] | |
− | + | #Take a black 96-well plate, and familiarize yourself with the plate map scheme at right: top two rows are to be loaded with wild-type IPC, next two rows are to be loaded with your X#Z mutant IPC, and the final row is to be loaded with water/BSA to serve as a blank/background row. | |
+ | #*The dark sides of the plate reduce "cross-talk" (''i.e.'', light leakage) between samples in adjacent wells, another potential contribution to error. | ||
+ | #Aliquot your wild-type protein to your plate. Use your P200 pipet to add 30 μL of protein (per well) to rows A and B of your plate. | ||
+ | #Aliquot you X#Z mutant IPC to your plate. Use your P200 pipet and add 30 μL of protein (per well) to rows C and D of your plate. | ||
+ | #Finally, add 30 μL of water with only 0.1% BSA (no IPC) to row 5(E) of your plate. | ||
+ | #The calcium solutions are at the front bench in shared reservoirs. '''Carefully''' carry your plate to the front bench to add these solutions with the multi-channel pipet. | ||
+ | #Using shared reservoir #1 (lowest calcium concentration - actually 0 nM), add 30 μL to the top five rows in the first column of the plate. Discard the pipet tips. | ||
+ | #Now work your way from reservoirs #2 to #12 (highest calcium concentration), and from the left-hand to the right-hand columns on your plate. Be sure to use fresh pipet tips each time! If you do contaminate a solution, let the teaching faculty know so they can put out some fresh solution. Honesty about a mistake is far preferred here to affecting every downstream experiment. | ||
+ | #When you are done, alert the teaching faculty and you will be taken in small groups to measure the fluorescence values for your samples. | ||
− | + | ===Part 3: Fluorescence assay=== | |
− | + | ||
− | + | ||
− | + | ||
− | + | ||
− | + | ||
− | + | ||
− | + | ||
− | + | ||
− | + | ||
− | + | ||
− | + | ||
− | + | ||
− | + | ||
− | ' | + | #The BMC (BioMicro Center) has graciously agreed to let us use their plate reader. Walk over to building 68 with a member of the teaching staff. |
+ | #You will be shown how to set the excitation (485 nm) and emission (515 nm) wavelength on the plate reader to assay your protein. | ||
+ | #Your raw data will be posted on today's [[Talk:20.109(S16):Characterize protein expression (Day7)|Discussion page]] and emailed to you as a .txt file so you can begin your analysis. | ||
− | + | You will analyze your calcium titration assay data in two steps. First, you will get a rough feel for how your mutant changed (or didn't) compared to wild-type IPC by plotting the two replicate values and their average, in both raw and processed form. Second, you will take the average processed values and plug them into some <small>MATLAB</small> code that will more precisely tell you the affinity and cooperativity of each protein with respect to calcium. | |
− | + | ===Part 1: Titration curve in Excel and first estimate of ''K<sub>d</sub>''=== | |
− | + | ||
− | + | ||
− | + | ||
− | + | ||
− | + | ||
− | + | ||
− | + | ||
− | + | ||
− | + | ||
− | + | ||
− | + | ||
− | + | Today you will analyze the fluorescence data that you got last time. Begin by analyzing the wild-type protein as a check on your work (your curve should resemble Nagai's Figure 3L), and then move on to your mutant samples. If you are not familiar with manipulations in Excel, use the ''Help'' menu or ask the teaching faculty for assistance. | |
− | + | #Open an Excel file for your data analysis. Begin by making a column of the free calcium concentrations present in your twelve test solutions. Assuming a 1:1 dilution of protein with calcium, the final concentrations are: 0 nM, 8.5 nM, 19 nM, 32.5 nM, 50 nM, 75 nM, 112.5 nM, 175 nM, 301 nM, 675 nM, 1.505 μM, 19.5 μM. Be sure to convert all concentrations to the same units.<br> | |
+ | #Now open the text file containing your raw data as a tab-delimited file in Excel (you can download the file from the [[Talk:20.109(S16):Characterize protein expression (Day7) | M1D7 Discussion]] page). Convert the row-wise data to column-wise data (using ''Paste Special'' → ''Transpose''), and transfer each column to your analysis file. Add column headers to indicate which protein is which, and analyze each replicate separately for now. Also include a column of your control samples that did not contain protein. | ||
+ | #Begin by calculating the average of your blank samples, and bold this number for easy reference. It is the background fluorescence present in the calcium solutions and should be quite low. If necessary, subtract this background value from each of your raw data values. It may help to have a 6-column series called “RAW”, and another called “SUBTRACTED.” | ||
+ | #Next you should normalize your data. The maximum and minimum fluorescence values for a given titration series should be defined as 100% and 0% fluorescence, respectively, and every other fluorescence value should be expressed as a percentage in between. Think about how to mathematically express these conditions. | ||
+ | #*First calculate the percent fluorescence for both replicates. Then make a new column and calculate the average percentage as well. | ||
+ | #*Alternatively, average your data first, and then normalize the average data. How do the "average then normalize" and the "normalize then average" curves compare? Which one will you include in your Protein engineering summary? | ||
+ | #*If one data point seems really off from the other replicate and from the expected trend, you might consider it an outlier and delete it, especially if you have good reason to believe that there was a reason (error in pipetting, air bubble in that well) for the anomaly. Otherwise, you might be losing valuable information, and/or misleading anyone who tries to interpret your data. | ||
+ | #For each protein, plot this normalized data versus calcium concentration. Save these plots in case you want to include them in your report. | ||
+ | #*You might plot the two replicates as points and their average value as a dashed line (see [http://engineerbiology.org/wiki/20.109%28S16%29:Module_1 front page] of this module). | ||
+ | #Note down the approximate inflection points of the curves, which should occur at half-saturation: these indicate the approximate values of the apparent <math>K_d</math> for each sample. | ||
− | ''' | + | ===Part 2: Improved estimate of ''K<sub>d</sub>'' using <small>MATLAB</small> modeling=== |
− | [[ | + | ====Preparation==== |
+ | #Download these three files: [[Media:F15 Fit Main.m | F15_Fit_Main]], [[Media:Fit_SingleKD.m| Fit_SingleKD]], and [[Media:Fit_KDn.m| Fit_KDn]]. Move them to the username/Documents/MATLAB folder on your computer. | ||
+ | # Double-click on the <small>MATLAB</small> icon to start up this software. | ||
+ | # The main window that opens is called the command window: here is where you run programs (or directly input commands) and view outputs. You can also see and access the command history, workspace, and current directory windows, but you likely won’t need to today. | ||
+ | # In the command window, type ''more on''; this command allows you to scroll through multi-page output (using the spacebar), such as help files. | ||
+ | # In addition to the command area, <small>MATLAB</small> comes with an editor. Click ''File'' → ''Open'' and select the program '''F15_Fit_Main'''. It has the .m extension and thus is executable by <small>MATLAB</small>. Read the introductory comments (the beginning of a comment is indicated by a % sign), and then input your fluorescence data. | ||
+ | # Read through the program, and as you encounter unfamiliar terms, return to the workspace and type ''help functionname''. Feel free to ask questions of the teaching faculty as well. | ||
+ | #* You might read about such built-in functions as ''logspace'' and ''nlinfit''. | ||
+ | #* You will also want to open and read '''Fit_SingleKD''' – a user-defined function called by '''F15_Fit_Main''' – in the <small>MATLAB</small> editor. | ||
+ | #* If you type ''help function'' you will learn the syntax for a function header. | ||
+ | #* Note that a dot preceeding an operator (such as A ./ B or A .* B) is a way of telling <small>MATLAB</small> to perform element-by-element rather than matrix algebra. | ||
+ | #* Also note that when a line of code is ''not'' followed by a semi-colon, the value(s) resulting from the operation will be displayed in the command window. | ||
− | + | ====Analysis==== | |
− | # | + | # Once you more-or-less follow Part 1 of the program, type '''F15_Fit_Main''' in the workspace, hit return to run the program, and consider the following questions: |
+ | #* Why must the fluorescence data be transformed (from ''S'' to ''Y'') prior to using in the model? | ||
+ | #* What <math>K_d</math> values are output in the command window, and how do they compare to the values you estimated from your Excel plots? | ||
+ | #* Figure 1 should display your wild type and mutant data points and model curves. How do they look in comparison to the curves you plotted in Excel? | ||
+ | #* Figure 2 should display the residuals (difference between data and model) for your three proteins. If the absolute values are low, this indicates good agreement between the model and the data numerically. Whether or not this is the case, another thing to look for is whether the residuals are evenly and randomly distributed about the zero-line. If there is a pattern to the errors, likely there is a systematic difference between the data and the model, and thus the model does not reflect the actual binding process well. What are the residuals like for each of your modeled proteins? | ||
+ | # Now move on to Part 2 of the '''F15_Fit_Main''' program. Part 2 also fits the data to a model with a single, ‘apparent’ value of <math>K_d</math>, but it allows for multiple binding sites and tests for cooperativity among them. The parameter used to measure cooperativity is called the Hill coefficient. A Hill coefficient of 1 indicates independent binding sites, while greater or lesser values reflect positive or negative cooperativity, respectively. Let the following questions guide you as you proceed: | ||
+ | #*Visually, which model appears to fit your wild-type data better (Fig. 3 ''vs.'' Fig. 1)? Your mutant data? | ||
+ | #*Do the respective residuals support your qualitative assessment (Fig. 4 ''vs.'' Fig. 2)? | ||
+ | #*Numerically, how do the values of <math>K_d</math> compare for the two models? How does the value of ''n'' compare to the implicitly assumed value of 1 in Part 1? | ||
+ | #*Do you see changes in binding affinity and/or cooperativity between the wild-type and X#Z samples? Do they match your ''a priori'' predictions? | ||
+ | #*'''Don't forget to save any figures you want to use in your report!''' If the legends are covering up your data, you can simply move them over with your mouse. | ||
+ | # Finally, you can skim Part 3 of the '''F15_Fit_Main''' program. Make sure you update the range of the linear transition region for each IPC sample, but beyond this, don’t worry too much about the coding details; rather do read through the comments. | ||
+ | #* Look at Part 1 of Figure 5: are the binding curves asymptotic, sigmoidal, or other? What does this shape indicate? You can use the zoom button to get a closer look at part of the plot, or the ''axis'' command present in the code. (Don't worry too much about this question if it is unclear.) | ||
+ | #* Now look in the command window. What values of <math>K_d</math> and Hill coefficient (''n'') do you get for your three proteins? How do the <math>K_d</math>’s from Part 3 compare to the ones from Parts 1 and 2? Don’t be discouraged if your wild-type values do not exactly match Nagai’s work, or if there is variation between Parts 1, 2, and 3. | ||
+ | #*Comparing the model and data points by eye (Part 2 of Figure 5), do you think it is a good model for any of your proteins? If so, which ones? What experimental limitations might prevent Hill analysis from working well, especially for some mutants? | ||
+ | #*Why should only the transition region be analyzed in a Hill plot? | ||
+ | #* What is the relationship between slope and <math>K_d</math> and/or ''n'', and intercept and <math>K_d</math> and/or ''n''? | ||
+ | #If your mutant proteins are not well-described by any of the models so far, what kind of model(s) (qualitatively speaking) do you think might be useful? | ||
+ | #*Optional: If your data might be well-described by a model with two <math>K_d</math>'s (or if you are interesting in exploring some sample data that is), download and run [[Media:Fit_TwoKD.m | Fit_TwoKD]] and [[Media:Fit_TwoKD_Func.m | Fit_TwoKD_Func]]. | ||
− | + | ==Reagent list== | |
− | |||
− | |||
− | |||
− | |||
− | |||
− | |||
− | |||
− | |||
− | |||
− | |||
− | |||
− | |||
− | |||
− | |||
− | |||
− | |||
− | |||
− | |||
− | |||
− | |||
− | |||
− | |||
− | |||
− | |||
− | |||
− | |||
− | |||
− | |||
− | |||
− | |||
− | |||
− | |||
− | |||
− | |||
− | |||
− | |||
− | |||
− | |||
− | |||
− | |||
− | |||
− | |||
− | |||
− | |||
− | |||
− | |||
− | |||
− | |||
− | |||
− | |||
− | |||
− | |||
− | |||
− | |||
− | |||
− | |||
− | |||
− | |||
− | |||
− | |||
− | |||
− | |||
− | |||
− | |||
− | |||
− | |||
− | |||
− | |||
− | |||
− | |||
− | |||
− | |||
− | |||
− | |||
− | |||
− | |||
− | |||
− | |||
− | |||
− | |||
− | |||
− | |||
− | |||
− | |||
− | |||
− | |||
− | |||
− | |||
− | |||
− | |||
− | |||
− | |||
− | |||
− | |||
− | |||
− | |||
− | |||
− | |||
− | |||
− | |||
− | |||
− | + | *Calcium calibration kit from Life Technologies | |
− | + | **Zero free calcium buffer: 10 mM EGTA in 100 mM KCl, 30 mM MOPS, pH 7.2 | |
− | + | **39 μM free calcium buffer: 10 mM CaEGTA in 100 mM KCl, 30 mM MOPS, pH 7.2 | |
− | + | ||
− | + | ||
− | + | ||
− | + | ||
− | + | ||
− | + | ||
− | + | ||
− | + | ||
− | + | ||
− | + | ||
− | + | ||
− | + | *Thermo Scientific Varioskan Flash Spectral Scanning Multimode Reader | |
− | * | + | |
− | + | ||
− | + | ||
− | + | ||
− | + | ||
− | + | ||
− | + | ||
− | + | ||
− | + | ||
− | + | ||
− | + | ||
− | + | ||
− | + | ||
− | + | ||
− | + | ||
− | + | ||
− | + | ||
− | + | ||
==Navigation links== | ==Navigation links== | ||
− | Next day: [[20.109(S21):M3D4 | | + | Next day: [[20.109(S21):M3D4 |Design new IPC variant ]] <br> |
− | Previous day: [[20.109(S21):M3D2 | | + | Previous day: [[20.109(S21):M3D2 |Identify IPC mutations]] <br> |
Revision as of 15:17, 19 April 2021
Introduction
As evidenced by Nagai’s work, wild-type inverse pericam is not toxic to BL21(DE3)pLysS cells. Although it is unlikely that your point mutation will dramatically change this fact, in general a novel protein may turn out to be toxic. If this is the case, only very small amounts of protein are produced before the bacteria die. Keep in mind that overexpressing a single protein may come at the expense of producing proteins needed for survival, and will most likely cause cell death eventually; however, toxic proteins hasten this demise. Aberrant toxicity can sometimes be alleviated by reducing the culture temperature (e.g., to 30 °C).
Based on its fluorescence activity, wild-type inverse pericam allows proper folding of (cp)EYFP, and based on its response to calcium, it also allows calmodulin to fold. One problem you may encounter is that your mutant proteins will no longer fold correctly. Since you made mutations in the calcium sensor part of IPC, rather than the fluorescent part, it is unlikely that your protein will destroy EYFP fluorescence. However, a common problem with misfolded proteins is the formation of insoluble aggregates, due for instance to improperly exposed hydrophobic surfaces. Proteins can be purified from these aggregates – called inclusion bodies – but the process is more labor-intensive than for soluble proteins. (The proteins must be extracted under more harsh conditions than you will use next time, then purified under denaturing conditions, before finally attempting to renature the proteins.) Inclusion bodies sometimes form simply due to very high expression of the protein of interest, causing it to pass its solubility limit. This outcome can be prevented by lowering the culture temperature, the induction duration, the amount of IPTG, or the growth phase of the bacteria.
One final point to keep in mind is that not all proteins can be produced in bacteria. Eukaryotic proteins that require post-translational modifications (such as glycosylation) for activity require eukaryotic hosts (such as yeast, or the commonly used CHO – Chinese hamster ovary – cells). Sometimes eukaryote-derived proteins will be truncated or otherwise mistranslated by E. coli due to differential codon bias; errors in translation can be prevented by providing additional tRNAs to the culture or directly to the bacteria via plasmids. Despite all this complexity, prokaryotic hosts have been plenty good enough to produce proteins for certain therapies, notably the cytokine G-CSF. This cytokine is taken by patients needing to replenish their white blood cells (e.g., after chemotherapy), and sold as Neupogen by the company Amgen.
This is it, folks! Moment of truth. Time to find out how the proteins that you worked so hard to express, purify, and test really behave. Although you should be able to produce reasonable titration curves by following the example of Nagai, the introduction/review of binding constants below may help contextualize your analysis.
Let’s start by considering the simple case of a receptor-ligand pair that are exclusive to each other, and in which the receptor is monovalent. The ligand (L) and receptor (R) form a complex (C), which can be written
$ R + L \rightleftharpoons\ ^{k_f}_{k_r} C $
At equilibrium, the rates of the forward reaction (rate constant = $ k_f $) and reverse reaction (rate constant = $ k_r $) must be equivalent. Solving this equivalence yields an equilibrium dissociation constant $ K_d $, which may be defined either as $ k_r/k_f $, or as $ [R][L]/[C] $, where brackets indicate the molar concentration of a species. Meanwhile, the fraction of receptors that are bound to ligand at equilibrium, often called y or θ, is $ C/R_{TOT} $, where $ R_{TOT} $ indicates total (both bound and unbound) receptors. Note that the position of the equilibrium (i.e., y) depends on the starting concentrations of the reactants; however, $ K_d $ is always the same value. The total number of receptors $ R_{TOT} $= [C] (ligand-bound receptors) + [R] (unbound receptors). Thus,
$ \qquad y = {[C] \over R_{TOT}} \qquad = \qquad {[C] \over [C] + [R]} \qquad = \qquad {[L] \over [L] + [K_d]} \qquad $
where the right-hand equation was derived by algebraic substitution. If the ligand concentration is in excess of the concentration of the receptor, [L] may be approximated as a constant, L, for any given equilibrium. Let’s explore the implications of this result:
- What happens when L << $ K_d $?
- →Then y ~ $ L/K_d $, and the binding fraction increases in a first-order fashion, directly proportional to L.
- What happens when L >> $ K_d $?
- →In this case y ~1, so the binding fraction becomes approximately constant, and the receptors are saturated.
- What happens when L = $ K_d $?
- →Then y = 0.5, and the fraction of receptors that are bound to ligand is 50%. This is why you can read $ K_d $ directly off of the plots in Nagai’s paper (compare Figure 3 and Table 1). When y = 0.5, the concentration of free calcium (our [L]) is equal to $ K_d $. This is a great rule of thumb to know.
The figures below demonstrate how to read $ K_d $ from binding curves. You will find semilog plots (right) particularly useful today, but the linear plot (left) can be a helpful visualization as well. Keep in mind that every L value is associated with a particular equilbrium value of y, while the curve as a whole gives information on the global equilibrium constant $ K_d $.
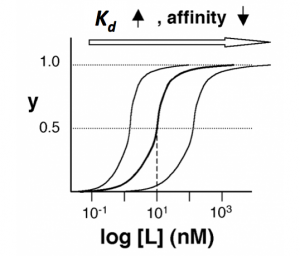
Of course, inverse pericam has multiple binding sites, and thus IPC-calcium binding is actually more complicated than the example above. The $ K_d $ reported by Nagai is called an ‘apparent $ K_d $’ because it reflects the overall avidity of multiple calcium binding sites, not their individual affinities for calcium. Normally, calmodulin has a low affinity (N-terminus) and a high affinity (C-terminus) pair of calcium binding sites. However, the E104Q mutant, which is the version of CaM used in inverse pericam, displays low affinity binding at both termini. Moreover, the Hill coefficient, which quantifies cooperativity of binding in the case of multiple sites, is reported to be 1.0 for inverse pericam. This indicates that inverse pericam behaves as if it were binding only a single calcium ion per molecule. Thus, wild-type IPC is well-described by a single apparent $ K_d $.
For any given mutant, things may be more complicated. Keep in mind that we are not directly measuring calcium binding, but instead are indirectly inferring it based on fluorescence (for both mutant and wild-type IPC). A change in fluorescence requires the participation not only of calcium, but also of M13. In addition to the four separate calcium binding sites in calmodulin, the M13 binding site influences apparent affinity and apparent cooperativity. In short, be careful about how you describe the meanings of our binding parameters in your reports.
Returning to the big picture: when you write your Protein engineering summary, be sure to consider how changes in both binding affinity and cooperativity (and even potentially raw fluorescence differences) can affect the practical utility of a sensor.
Protocols
Part 2: Prepare samples for titration curve
Tips for success
Take great care today to limit the introduction of bubbles in your samples. When expelling fluid, pipet slowly while touching the pipet tip against the bottom or side of the well.
Protocol
- Take a black 96-well plate, and familiarize yourself with the plate map scheme at right: top two rows are to be loaded with wild-type IPC, next two rows are to be loaded with your X#Z mutant IPC, and the final row is to be loaded with water/BSA to serve as a blank/background row.
- The dark sides of the plate reduce "cross-talk" (i.e., light leakage) between samples in adjacent wells, another potential contribution to error.
- Aliquot your wild-type protein to your plate. Use your P200 pipet to add 30 μL of protein (per well) to rows A and B of your plate.
- Aliquot you X#Z mutant IPC to your plate. Use your P200 pipet and add 30 μL of protein (per well) to rows C and D of your plate.
- Finally, add 30 μL of water with only 0.1% BSA (no IPC) to row 5(E) of your plate.
- The calcium solutions are at the front bench in shared reservoirs. Carefully carry your plate to the front bench to add these solutions with the multi-channel pipet.
- Using shared reservoir #1 (lowest calcium concentration - actually 0 nM), add 30 μL to the top five rows in the first column of the plate. Discard the pipet tips.
- Now work your way from reservoirs #2 to #12 (highest calcium concentration), and from the left-hand to the right-hand columns on your plate. Be sure to use fresh pipet tips each time! If you do contaminate a solution, let the teaching faculty know so they can put out some fresh solution. Honesty about a mistake is far preferred here to affecting every downstream experiment.
- When you are done, alert the teaching faculty and you will be taken in small groups to measure the fluorescence values for your samples.
Part 3: Fluorescence assay
- The BMC (BioMicro Center) has graciously agreed to let us use their plate reader. Walk over to building 68 with a member of the teaching staff.
- You will be shown how to set the excitation (485 nm) and emission (515 nm) wavelength on the plate reader to assay your protein.
- Your raw data will be posted on today's Discussion page and emailed to you as a .txt file so you can begin your analysis.
You will analyze your calcium titration assay data in two steps. First, you will get a rough feel for how your mutant changed (or didn't) compared to wild-type IPC by plotting the two replicate values and their average, in both raw and processed form. Second, you will take the average processed values and plug them into some MATLAB code that will more precisely tell you the affinity and cooperativity of each protein with respect to calcium.
Part 1: Titration curve in Excel and first estimate of Kd
Today you will analyze the fluorescence data that you got last time. Begin by analyzing the wild-type protein as a check on your work (your curve should resemble Nagai's Figure 3L), and then move on to your mutant samples. If you are not familiar with manipulations in Excel, use the Help menu or ask the teaching faculty for assistance.
- Open an Excel file for your data analysis. Begin by making a column of the free calcium concentrations present in your twelve test solutions. Assuming a 1:1 dilution of protein with calcium, the final concentrations are: 0 nM, 8.5 nM, 19 nM, 32.5 nM, 50 nM, 75 nM, 112.5 nM, 175 nM, 301 nM, 675 nM, 1.505 μM, 19.5 μM. Be sure to convert all concentrations to the same units.
- Now open the text file containing your raw data as a tab-delimited file in Excel (you can download the file from the M1D7 Discussion page). Convert the row-wise data to column-wise data (using Paste Special → Transpose), and transfer each column to your analysis file. Add column headers to indicate which protein is which, and analyze each replicate separately for now. Also include a column of your control samples that did not contain protein.
- Begin by calculating the average of your blank samples, and bold this number for easy reference. It is the background fluorescence present in the calcium solutions and should be quite low. If necessary, subtract this background value from each of your raw data values. It may help to have a 6-column series called “RAW”, and another called “SUBTRACTED.”
- Next you should normalize your data. The maximum and minimum fluorescence values for a given titration series should be defined as 100% and 0% fluorescence, respectively, and every other fluorescence value should be expressed as a percentage in between. Think about how to mathematically express these conditions.
- First calculate the percent fluorescence for both replicates. Then make a new column and calculate the average percentage as well.
- Alternatively, average your data first, and then normalize the average data. How do the "average then normalize" and the "normalize then average" curves compare? Which one will you include in your Protein engineering summary?
- If one data point seems really off from the other replicate and from the expected trend, you might consider it an outlier and delete it, especially if you have good reason to believe that there was a reason (error in pipetting, air bubble in that well) for the anomaly. Otherwise, you might be losing valuable information, and/or misleading anyone who tries to interpret your data.
- For each protein, plot this normalized data versus calcium concentration. Save these plots in case you want to include them in your report.
- You might plot the two replicates as points and their average value as a dashed line (see front page of this module).
- Note down the approximate inflection points of the curves, which should occur at half-saturation: these indicate the approximate values of the apparent $ K_d $ for each sample.
Part 2: Improved estimate of Kd using MATLAB modeling
Preparation
- Download these three files: F15_Fit_Main, Fit_SingleKD, and Fit_KDn. Move them to the username/Documents/MATLAB folder on your computer.
- Double-click on the MATLAB icon to start up this software.
- The main window that opens is called the command window: here is where you run programs (or directly input commands) and view outputs. You can also see and access the command history, workspace, and current directory windows, but you likely won’t need to today.
- In the command window, type more on; this command allows you to scroll through multi-page output (using the spacebar), such as help files.
- In addition to the command area, MATLAB comes with an editor. Click File → Open and select the program F15_Fit_Main. It has the .m extension and thus is executable by MATLAB. Read the introductory comments (the beginning of a comment is indicated by a % sign), and then input your fluorescence data.
- Read through the program, and as you encounter unfamiliar terms, return to the workspace and type help functionname. Feel free to ask questions of the teaching faculty as well.
- You might read about such built-in functions as logspace and nlinfit.
- You will also want to open and read Fit_SingleKD – a user-defined function called by F15_Fit_Main – in the MATLAB editor.
- If you type help function you will learn the syntax for a function header.
- Note that a dot preceeding an operator (such as A ./ B or A .* B) is a way of telling MATLAB to perform element-by-element rather than matrix algebra.
- Also note that when a line of code is not followed by a semi-colon, the value(s) resulting from the operation will be displayed in the command window.
Analysis
- Once you more-or-less follow Part 1 of the program, type F15_Fit_Main in the workspace, hit return to run the program, and consider the following questions:
- Why must the fluorescence data be transformed (from S to Y) prior to using in the model?
- What $ K_d $ values are output in the command window, and how do they compare to the values you estimated from your Excel plots?
- Figure 1 should display your wild type and mutant data points and model curves. How do they look in comparison to the curves you plotted in Excel?
- Figure 2 should display the residuals (difference between data and model) for your three proteins. If the absolute values are low, this indicates good agreement between the model and the data numerically. Whether or not this is the case, another thing to look for is whether the residuals are evenly and randomly distributed about the zero-line. If there is a pattern to the errors, likely there is a systematic difference between the data and the model, and thus the model does not reflect the actual binding process well. What are the residuals like for each of your modeled proteins?
- Now move on to Part 2 of the F15_Fit_Main program. Part 2 also fits the data to a model with a single, ‘apparent’ value of $ K_d $, but it allows for multiple binding sites and tests for cooperativity among them. The parameter used to measure cooperativity is called the Hill coefficient. A Hill coefficient of 1 indicates independent binding sites, while greater or lesser values reflect positive or negative cooperativity, respectively. Let the following questions guide you as you proceed:
- Visually, which model appears to fit your wild-type data better (Fig. 3 vs. Fig. 1)? Your mutant data?
- Do the respective residuals support your qualitative assessment (Fig. 4 vs. Fig. 2)?
- Numerically, how do the values of $ K_d $ compare for the two models? How does the value of n compare to the implicitly assumed value of 1 in Part 1?
- Do you see changes in binding affinity and/or cooperativity between the wild-type and X#Z samples? Do they match your a priori predictions?
- Don't forget to save any figures you want to use in your report! If the legends are covering up your data, you can simply move them over with your mouse.
- Finally, you can skim Part 3 of the F15_Fit_Main program. Make sure you update the range of the linear transition region for each IPC sample, but beyond this, don’t worry too much about the coding details; rather do read through the comments.
- Look at Part 1 of Figure 5: are the binding curves asymptotic, sigmoidal, or other? What does this shape indicate? You can use the zoom button to get a closer look at part of the plot, or the axis command present in the code. (Don't worry too much about this question if it is unclear.)
- Now look in the command window. What values of $ K_d $ and Hill coefficient (n) do you get for your three proteins? How do the $ K_d $’s from Part 3 compare to the ones from Parts 1 and 2? Don’t be discouraged if your wild-type values do not exactly match Nagai’s work, or if there is variation between Parts 1, 2, and 3.
- Comparing the model and data points by eye (Part 2 of Figure 5), do you think it is a good model for any of your proteins? If so, which ones? What experimental limitations might prevent Hill analysis from working well, especially for some mutants?
- Why should only the transition region be analyzed in a Hill plot?
- What is the relationship between slope and $ K_d $ and/or n, and intercept and $ K_d $ and/or n?
- If your mutant proteins are not well-described by any of the models so far, what kind of model(s) (qualitatively speaking) do you think might be useful?
- Optional: If your data might be well-described by a model with two $ K_d $'s (or if you are interesting in exploring some sample data that is), download and run Fit_TwoKD and Fit_TwoKD_Func.
Reagent list
- Calcium calibration kit from Life Technologies
- Zero free calcium buffer: 10 mM EGTA in 100 mM KCl, 30 mM MOPS, pH 7.2
- 39 μM free calcium buffer: 10 mM CaEGTA in 100 mM KCl, 30 mM MOPS, pH 7.2
- Thermo Scientific Varioskan Flash Spectral Scanning Multimode Reader
Next day: Design new IPC variant